Rui Dias – School of Business and Administration, Polytechnic Institute of Setúbal, Portugal; CEFAGE-UE, IIFA, University of Évora, Portugal
Nicole Horta – School of Business and Administration, Polytechnic Institute of Setúbal, Portugal
Catarina Revez – School of Business and Administration, Polytechnic Institute of Setúbal, Portugal
Paula Heliodoro – School of Business and Administration, Polytechnic Institute of Setúbal, Portugal
Paulo Alexandre – School of Business and Administration, Polytechnic Institute of Setúbal, Portugal
Keywords: Cryptocurrency markets;
Efficient market hypothesis;
Market efficiency
DOI: https://doi.org/10.31410/ERAZ.2022.87
Abstract: When compared to traditional financial markets, cryptocurrencies were seen as assets with minimal correlations. However, because this continually expanding financial market is marked by substantial volatility and strong price movements over a short period, developing an accurate and reliable forecasting model is deemed crucial for portfolio management and optimization. Given the relevance of cryptocurrencies in the global economy, it is important to determine if Bitcoin (BTC) becomes more predictable as investors adopt more aggressive trading positions. We examine BTC over the period from May 15th, 2021, to April 14th, 2022 (8676-time data), using intraday (hourly) time scales. The results reveal that the random walk hypothesis is rejected at lags of 3 to 16 days, while we see that the BTC market tends toward efficiency (see the evolution between lags of 16 and 2). These findings reveal that, given the uncertainty in the global economy in 2022, namely the Russian invasion of Ukraine, the BTC market shows values of the variance ratios close to unity, implying that it is, apparently, not predictable and that the residuals are not autocorrelated in time. In addition, the results of the Detrended Fluctuation Analysis (DFA) exponent show that this market does not exhibit characteristics of (in) efficiency in its weak form. In other words, this market does not have persistent and mean-reverting properties, thus validating the results of Wright’s Rankings and Signs variance test.
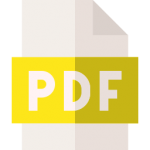
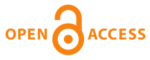
8th International Scientific ERAZ Conference – ERAZ 2022 – Conference Proceedings: KNOWLEDGE BASED SUSTAINABLE DEVELOPMENT, Online-Virtual (Prague, Czech Republic), May 26, 2022
ERAZ Conference Proceedings published by: Association of Economists and Managers of the Balkans – Belgrade, Serbia
ERAZ conference partners: Faculty of Economics and Business, Mediterranean University, Montenegro; University of National and World Economy – Sofia, Bulgaria; Faculty of Commercial and Business Studies – Celje, Slovenia; AMBIS University, Prague – Czech Republic; Faculty of Applied Management, Economics and Finance – Belgrade, Serbia
ERAZ Conference 2022 Conference Proceedings: ISBN 978-86-80194-60-8, ISSN 2683-5568, DOI: https://doi.org/10.31410/ERAZ.2022
Creative Commons Non Commercial CC BY-NC: This article is distributed under the terms of the Creative Commons Attribution-Non-Commercial 4.0 License (https://creativecommons.org/licenses/by-nc/4.0/) which permits non-commercial use, reproduction and distribution of the work without further permission.
Sugested citation
Dias, R., Horta, N., Revez, C., Heliodoro, P., & Alexandre, P. (2022). The Evolution of the Cryptocurrency Market Is Trending toward Efficiency? In V. Bevanda (Ed.), ERAZ Conference – Knowlegde Based Sustainable Development: Vol 8. Conference Proceedings (pp. 87-94). Association of Economists and Managers of the Balkans. https://doi.org/10.31410/ERAZ.2022.87
References
Chibane, M., & Janson, N. (2020). Do Bitcoin Stylized Facts Depend on Geopolitical Risk? SSRN Electronic Journal. https://doi.org/10.2139/ssrn.3530020
Dias, R., Heliodoro, P., & Alexandre, P. (2020). Efficiency of Asean-5 Markets: An Detrended Fluctuation Analysis. Mednarodno Inovativno Poslovanje = Journal of Innovative Business and Management, 12(2), 13–19. https://doi.org/10.32015/jibm.2020.12.2.13-19
Dias, R., Heliodoro, P., Alexandre, P., Santos, H., & Farinha, A. (2021). Long memory in stock returns: Evidence from the Eastern European markets. SHS Web of Conferences, 91. https://doi.org/10.1051/shsconf/20219101029
Dias, R., Pereira, J. M., & Carvalho, L. C. (2022). Are African Stock Markets Efficient? A Comparative Analysis Between Six African Markets, the UK, Japan and the USA in the Period of the Pandemic. Naše Gospodarstvo/Our Economy, 68(1), 35–51. https://doi.org/10.2478/ngoe-2022-0004
Dias, R. T., Pardal, P., Santos, H., & Vasco, C. (2021). Testing the Random Walk Hypothesis for Real Exchange Rates. June, 304–322. https://doi.org/10.4018/978-1-7998-6926-9.ch017
Dickey, D., & Fuller, W. (1981). Likelihood ratio statistics for autoregressive time series with a unit root. Econometrica, 49(4), 1057–1072. https://doi.org/10.2307/1912517
Dimitrova, V., Fernández-Martínez, M., Sánchez-Granero, M. A., & Segovia, J. E. T. (2019). Some comments on Bitcoin market (in)efficiency. PLoS ONE, 14(7). https://doi.org/10.1371/journal.pone.0219243
Fang, F., Ventre, C., Basios, M., Kanthan, L., Martinez-Rego, D., Wu, F., & Li, L. (2022). Cryptocurrency trading: a comprehensive survey. In Financial Innovation (Vol. 8, Issue 1). https://doi.org/10.1186/s40854-021-00321-6
Guedes, E. F., Santos, R. P. C., Figueredo, L. H. R., Da Silva, P. A., Dias, R. M. T. S., & Zebende, G. F. (2022). Efficiency and Long-Range Correlation in G-20 Stock Indexes: A Sliding Windows Approach. Fluctuation and Noise Letters. https://doi.org/10.1142/S021947752250033X
Hamayel, M. J., & Owda, A. Y. (2021). A Novel Cryptocurrency Price Prediction Model Using GRU, LSTM and bi-LSTM Machine Learning Algorithms. AI, 2(4). https://doi.org/10.3390/ai2040030
Jarque, C. M., & Bera, A. K. (1980). Efficient tests for normality, homoscedasticity and serial independence of regression residuals. Economics Letters, 6(3), 255–259. https://doi.org/10.1016/0165-1765(80)90024-5
Kakinaka, S., & Umeno, K. (2021). Exploring asymmetric multifractal cross-correlations of price–volatility and asymmetric volatility dynamics in cryptocurrency markets. Physica A: Statistical Mechanics and Its Applications, 581. https://doi.org/10.1016/j.physa.2021.126237
Kristoufek, L. (2018). On Bitcoin markets (in)efficiency and its evolution. Physica A: Statistical Mechanics and Its Applications, 503, 257–262. https://doi.org/10.1016/j.physa.2018.02.161
Krückeberg, S., & Scholz, P. (2020). Decentralized Efficiency? Arbitrage in Bitcoin Markets. Financial Analysts Journal, 76(3), 135–152. https://doi.org/10.1080/0015198X.2020.1733902
Kwiatkowski, D., Phillips, P. C. B., Schmidt, P., & Shinb, Y. (1992). Testing the null hypothesis of stationary against the alternative of a unit root. Journal of Econometrics, 54(1), 159– 178. https://doi.org/http://dx.doi.org/10.1016/0304-4076(92)90104-Y
Perron, P., & Phillips, P. C. B. (1988). Testing for a Unit Root in a Time Series Regression. Biometrika, 2(75), 335–346. https://doi.org/10.1080/07350015.1992.10509923
Shrestha, K. (2021). Multifractal Detrended Fluctuation Analysis of Return on Bitcoin*. In International Review of Finance (Vol. 21, Issue 1). https://doi.org/10.1111/irfi.12256
Wright, J. H. (2000). Alternative variance-ratio tests using ranks and signs. Journal of Business and Economic Statistics. https://doi.org/10.1080/07350015.2000.10524842
Wu, X., Wu, L., & Chen, S. (2022). Long memory and efficiency of Bitcoin during COVID-19. Applied Economics, 54(4). https://doi.org/10.1080/00036846.2021.1962513
Zebende, G. F., Santos Dias, R. M. T., & de Aguiar, L. C. (2022). Stock market efficiency: An intraday case of study about the G-20 group. Heliyon, 8(1), e08808. https://doi.org/10.1016/j.heliyon.2022.e08808