Mikhail Pomazanov – Higher School of Economics National Research University, Main campus: 20 Myasnitskaya Ulitsa, Moscow,
Russia
DOI: https://doi.org/10.31410/ERAZ.S.P.2020.11
6th International Conference – ERAZ 2020 – KNOWLEDGE BASED SUSTAINABLE DEVELOPMENT, Online/virtual, May 21, 2020, SELECTED PAPERS
Published by: Association of Economists and Managers of the Balkans – Belgrade, Serbia
Conference partners: Faculty of Economics and Business, Mediterranean University, Montenegro; University of National and World Economy – Sofia, Bulgaria; Faculty of Commercial and Business Studies – Celje, Slovenia; Faculty of Applied Management, Economics and Finance – Belgrade, Serbia
ISBN 978-86-80194-34-9, ISSN 2683-5568, DOI: https://doi.org/10.31410/ERAZ.
Abstract
This paper presents non-classical models for estimating and forecasting COVID-19 pandemic
indices. These models have been successfully tested on country data where the pandemic is nearing
completion. In particular, an effective algorithm for mortality index evaluation is also presented. This
index is usually replaced by more simple estimates such as, for instance, „the number of deaths divided
by the number of infected”; however, while the virus is at the stage of its rapid distribution, such superficial
approaches are incorrect. Model indicators of the infection itself allow us to predict not only the
apogee of the epidemic and the end of the quarantine period, but also the maximum number of infected
people in some country (continent) during the height of the epidemic.
The second part of the paper is devoted to an attempt to build regression models to explain (with using
100+ country socio-economic indicators taken from the World Bank data) the behavior of the epidemic
spread indices. It is shown that the maximum number of infected people in the country is well predicted
(R-square is close to 90%); and, moreover, migration indicators and the number of international air
take-offs are effective regressors. Other indicators, for example, the mortality index, are difficultly
modeled; nevertheless, it has a significant relationship with socio-economic factors.
The presented paper might be valuable for making effective decisions to forestall some future pandemics
or even the „second wave” of COVID-19.
Key words
COVID-19, Pandemic, Modeling, Socio-economic indicators.
References
Breusch T. S., Pagan A. R. (1979). A Simple Test for Heteroskedasticity and Random Coefficient
Variation”. Econometrica. 47 (5): 1287–1294. doi:10.2307/1911963. JSTOR 1911963. MR
0545960.
Choisy M., Guégan J.-F., and Rohani P. (2007) Mathematical Modeling of Infectious Diseases
Dynamics. Chapter 22, Encyclopedia of Infectious Diseases: Modern Methodologies, by
M. Tibayrenc. Copyright © 2007 John Wiley & Sons, Inc.
Ghani C. et al. (2005) Methods for Estimating the Case Fatality Ratio for a Novel, Emerging
Infectious Disease. American Journal of Epidemiology, Volume 162, Issue 5, 1 September
2005, Pages 479–486, https://doi.org/10.1093/aje/kwi230
Hastie T. J., Tibshirani R. J. (1990) Generalized Additive Models. — Chapman and Hall, ISBN
0-412-34390-8.
Herbert W., Hethcote (1989) Three Basic Epidemiological Models. Applied Mathematical Ecology.
pp 119-144. Editors Simon A. Levin Thomas G. Hallam Louis J. Gross. Springer-Verlag
Berlin Heidelberg DOI https://doi.org/10.1007/978-3-642-61317-3
Johns Hopkins CSSE (2019) Novel Coronavirus COVID-19 (2019-nCoV) Data Repository,
https://github.com/CSSEGISandData/2019-nCoV
The World Bank. Indicators. https://data.World Bank.org/indicator?tab=all
Volterra, V. (1976) The Mathematical Theory of the Struggle for Existence. Science, Moscow,
288.
WHO Situation Reports. Number of Cases and Deaths in Guinea, Liberia, and Sierra Leone
during the 2014-2016 West Africa Ebola Outbreak, https://www.cdc.gov/vhf/ebola/history/
2014-2016-outbreak/case-counts.html
WHO. Coronavirus disease (COVID-2019) situation reports. https://www.who.int/emergencies/
diseases/novel-coronavirus-2019/situation-reports
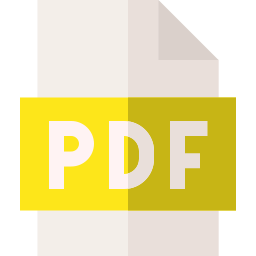
DOWNLOAD